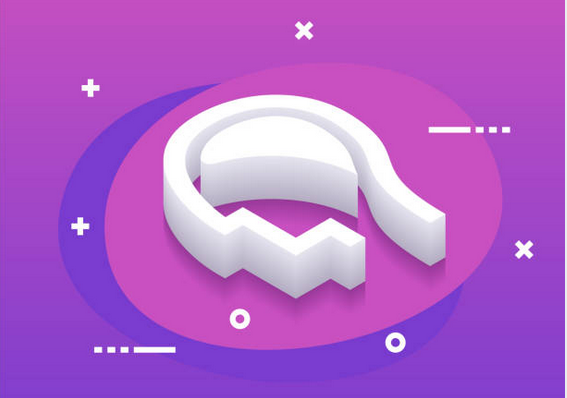
Dynamic Risk & Opportunity Based Profiling
Customer profiling has been constantly gaining in importance over the past few years, driven mainly by two independent forces. On one hand, regulators worldwide require financial institutions to do regular assessments of their customer and business relationships based on the so-called risk-based approach. On the other hand, more and more financial institutions realise the value and the potential of customer profiling for analytical CRM purposes in areas such as customer retention, cross and up-selling, revenue scoring or best next action. Surprisingly, even financial institutions that do both rarely apply this analysis in a uniform, consistent and integrative way across all areas of their organisation, but rather in isolation, in an inconsistent and limited manner.
The advantages of a truly uniform, consistent and integrative, 360° analytical customer view are more than obvious in terms of insight gained, completeness, accuracy, synergies, costs, infrastructure, data and resources. A simple and adequate linear regression or a decision tree analysis applied in isolation in one of these areas can turn into a precious source of hidden behavioural patterns when enriched with additional dimensions, analysed and considered from a multidimensional perspective in a unified and consistent manner.
In such cases, attributes that are completely static, usually irrelevant or even ignored suddenly become game-changers for a while, before disappearing again and going back to ‘normality’. But what is ‘normal’? How stable is this ‘normal’ nowadays? And for how long? Can you really reflect the complexity of the outside world by applying some ‘R’ or ‘PYTHON’ algorithms to a bunch of data targeting an isolated problem or question? How much subjectivity is involved in the selection of these algorithms, their configuration and parameterisation? Are you reliant on the skills and availability of an almost unaffordable quant for all that?
Clear and obvious answers
We talk about sophisticated data analytics approaches, debating and philosophising about what is ML and what is AI, riding on the AI wave, dazzled by buzzwords. At the same time, we seem to have missed the forest for the trees by focusing on isolated issues and silos rather than addressing complexity in a unified and consistent way. And why? Because it is easier, more comfortable and convenient. Our world is very much structured in silos and our thinking is often structured this way too. When we are confronted with complexity, we tend to simplify it or even ignore it.
Let’s take a step back, look at the big picture and reflect. Would you approach a customer with an offer for another banking product because your aCRM cross and up-selling analysis are predicting this right now, knowing that his creditworthiness is not great, knowing that he comes up in adverse media searches in relation to some negative news and that he is likely to be involved in money laundering and tax evasion? Probably not. Would you be interested to know that in addition to all that, a customer may be grossly negligent with regards to his cyber threat protection, exposing him and you to serious integrity risks, or that you might even be on the verge of losing him as a customer because of that, or because of a combination of reasons? I assume you would. Would you like to know how the current developments of the financial markets impact this specific customer’s profile with all his portfolios, exposures, risks and opportunities? I guess so. And do you think your customer would be interested in receiving competent and well-founded advice from you on what all that would mean from a tax or cross-border perspective if he were to change his country of residence? Yes, he definitely would. The answers seem to be clear and obvious, but nevertheless we continue to fall into these traps of irrational behaviour in applying isolated views, looking at these issues from one simplified perspective.
It is not difficult to move away from these problems though. Dynamic risk and opportunity based profiling is an integral, unified and consistent way of applying myriads of advanced ML and AI hybrid approaches in a contextual and situation-specific manner. Based on all available static and dynamic customer data, internal and external intelligence, it is not only focused on addressing one particular question or issue in isolation but provides a 360° analytical view of the customer, on his entire profile, with all his risks, threats and opportunities simultaneously. And of course, its purpose is not just to fulfil the requirements of the revised Swiss Anti-Money Laundering Act, to tick the compliance checkbox and to sigh with relief. It goes much further, taking into account the safety, accuracy and profitability of your daily decisions, reducing the risks and the threats, avoiding or reducing losses, and maximising profits. |
Nikolaï Tsenov
Product Manager Data Analytics
Finnova AG